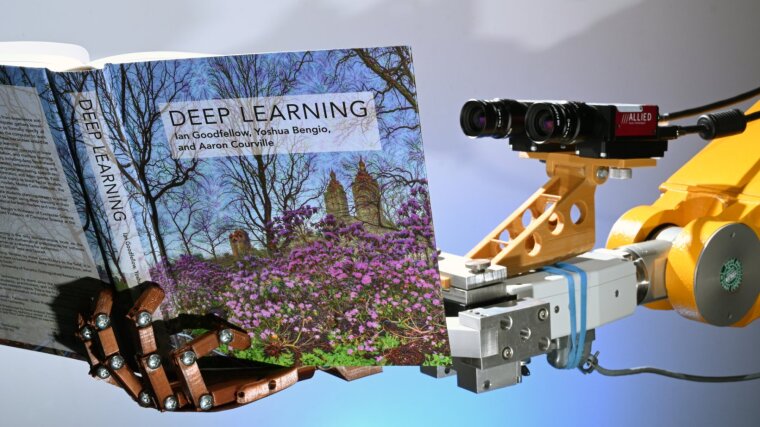
Status: active
Description
Machine learning (ML) is becoming an increasingly important scientific area supporting decision making and knowledge generation and is creating a high impact on several fields including healthcare, education, and many more. With this, it also becomes more and more important that the results of ML experiments are reproducible.
The goal of this proposed project is to enable reproducibility and interoperability of end-to-end ML pipelines via the development and exploitation of semantic annotations. The provenance information along with the semantic annotations will be used to make ML experiments more comparable, thus having a basis to judge progress of the field. The aim of the overall project is the application of semantic technologies to ML pipelines for efficient provenance capture, representation, query, comparison, and visualization to enable reproducibility and interoperability. The project is funded through the IMPULSE program of Friedrich-Schiller University Jena.
Member: Former Member: |